Machine Learning Basics
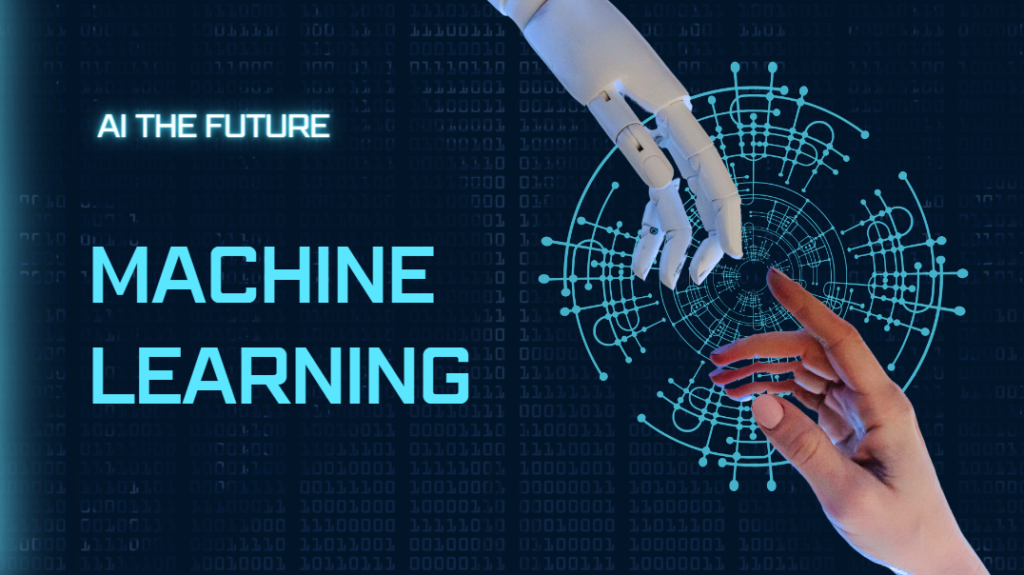
From Sci-Fi Fantasy to Everyday Reality
Imagine a world where computers learn and adapt like humans. No, this isn’t a scene straight out of a sci-fi movie; it’s the very core of machine learning (ML), a rapidly evolving field that’s transforming our world in countless ways.
But what exactly is machine learning? Let’s ditch the technical jargon and break it down in a way that makes sense, even if you’re new to the concept.
Learning Like a Boss: Unveiling the Magic Behind Machine Learning
Think about how you learned to ride a bike. You started wobbly, maybe even fell a few times, but with practice, you got better at balancing, steering, and pedaling. Machine learning works in a similar way. We feed machines massive amounts of data, kind of like giving them training wheels, and they use complex algorithms to identify patterns and relationships within that data. Over time, they become better at recognizing those patterns and even predicting future outcomes, all without needing explicit programming for every single situation.
Here’s a cool analogy: Imagine you’re training a friend to identify different types of dogs. You show them pictures of poodles, German shepherds, and pugs, pointing out the unique features of each breed. The more pictures they see, the better they become at recognizing different dogs on their own. Machine learning works on the same principle, except with much more complex data and tasks.
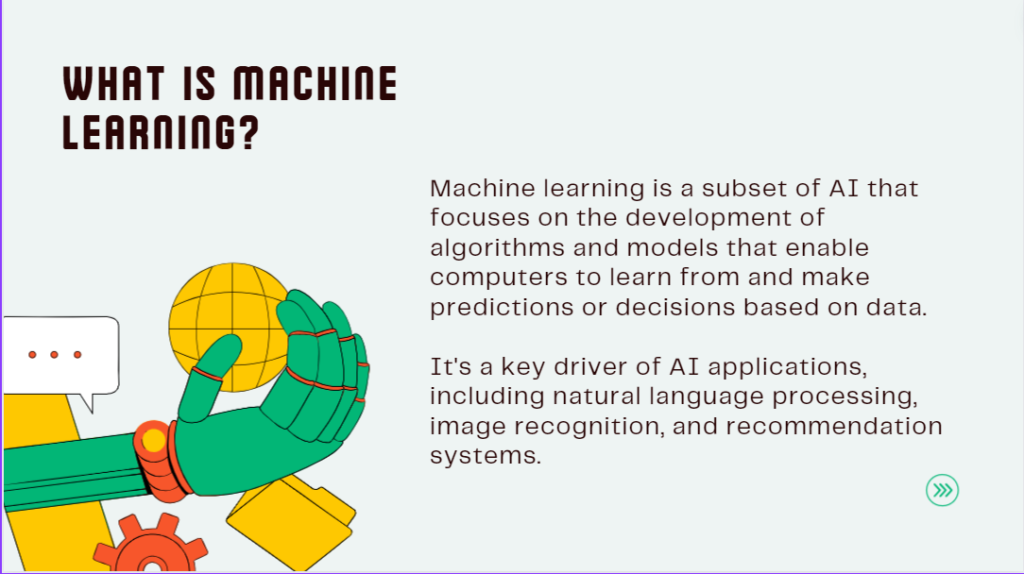
The Many Faces of Machine Learning:
Different Approaches for Different Problems
Machine learning isn’t a one-size-fits-all solution. There are different approaches used for different types of problems. Let’s explore some of the most common ones:
- Supervised Learning: This is kind of like having a teacher guide you. You provide the machine with labeled data, where each piece of data has a specific outcome already attached. For example, you might feed an algorithm thousands of emails labeled “spam” and “not spam.” By analyzing this data, the algorithm learns to identify spam emails on its own in the future.
- Unsupervised Learning: Now, imagine exploring a new city without a map. That’s unsupervised learning. You provide the machine with unlabeled data, and it has to find patterns and hidden structures within that data on its own. For example, an unsupervised learning algorithm might analyze customer purchase data and identify hidden trends, helping businesses understand their customer base better.
- Reinforcement Learning: This is where things get a bit more interactive. Imagine training a dog with treats. In reinforcement learning, the machine learns through trial and error, receiving rewards for good decisions and penalties for bad ones. A good example is how self-driving cars learn to navigate by receiving positive feedback for staying within the lane and negative feedback for getting too close to other vehicles.
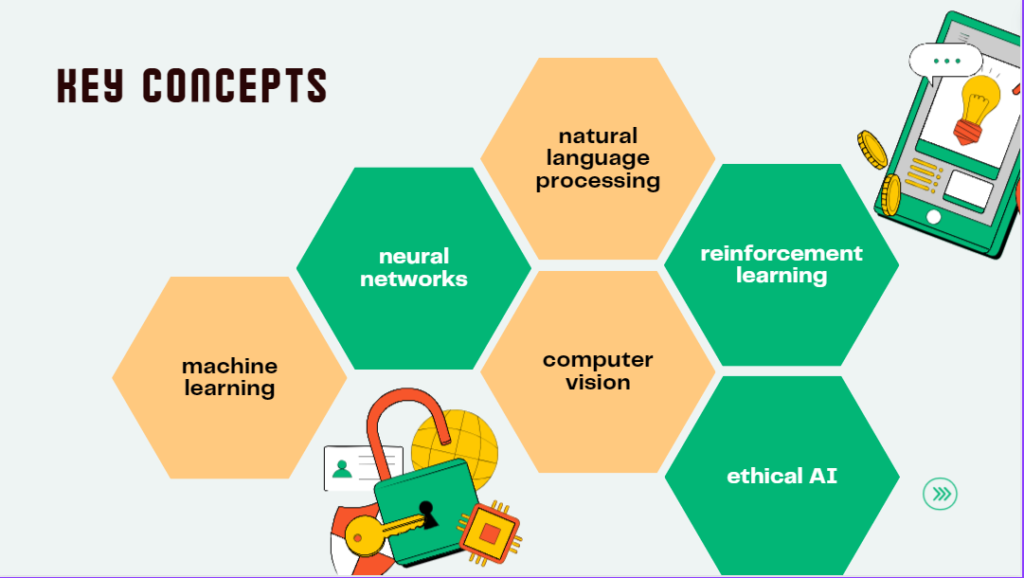
The Real-World Impact of Machine Learning
Machine learning is no longer confined to research labs. It’s already woven seamlessly into the fabric of our daily lives.
Here are just a few ways it’s making a difference:
- Making Your Life Easier: Ever scrolled through your social media feed and felt like it was reading your mind? That’s machine learning algorithms at work, recommending content they think you’ll like based on your past activity. Similarly, online shopping platforms recommend products based on your purchase history, making your shopping experience smoother.
- Revolutionizing Entertainment: Machine learning powers the recommendation engines on streaming services, suggesting movies and shows you might enjoy. It’s also used in video games to create realistic and dynamic opponents that adapt to your playing style.
- Boosting Business Efficiency: From targeted advertising to fraud detection, machine learning is a game-changer for businesses. Algorithms can analyze customer data to predict buying patterns, personalize marketing campaigns, and even identify fraudulent transactions before they happen.
- Shaping the Future of Tech: From self-driving cars to facial recognition software, machine learning is at the forefront of innovation. It’s constantly evolving, pushing the boundaries of what’s possible and shaping the future of technology.
The Road Ahead:
Challenges and Opportunities in the World of Machine Learning
While machine learning holds immense potential, it’s not without its challenges. Here are a few things to keep in mind:
- Data Bias: Machine learning algorithms are only as good as the data they’re trained on. If the data is biased, the algorithms will inherit that bias. This can lead to discriminatory outcomes, making it crucial to ensure fairness and inclusivity in data collection and algorithm design.
- The Black Box Problem: Sometimes, it’s difficult to understand how complex machine learning algorithms arrive at their decisions. This lack of transparency can raise concerns about accountability and fairness, especially in areas like facial recognition software used by law enforcement.
The Human Factor: Machine learning is a powerful tool, but it’s not a replacement for human intelligence and judgment. It’s essential to strike a balance, utilizing machine learning to automate tasks and improve efficiency while reserving critical decision-making and ethical considerations for humans.
Despite the challenges, the future of machine learning is brimming with opportunities.
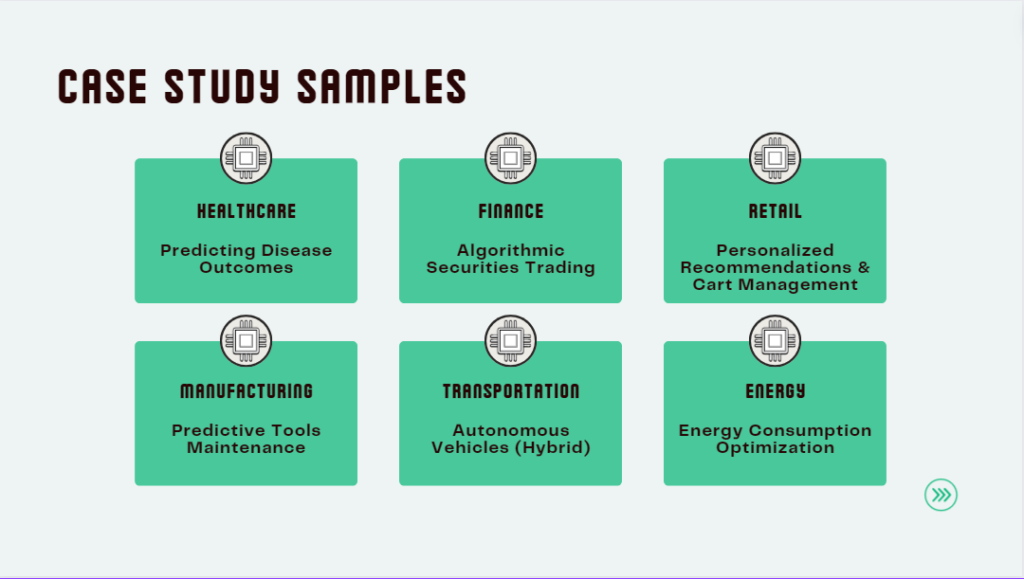
Here are some exciting possibilities on the horizon:
- Personalized Healthcare: Machine learning can analyze medical data to personalize treatment plans, predict disease outbreaks, and even assist in drug discovery. Imagine a future where doctors have AI-powered tools at their disposal to provide more accurate diagnoses and personalized care.
- Combating Climate Change: Machine learning can be used to analyze climate data, predict extreme weather events, and develop more sustainable solutions. It can also optimize energy consumption and identify areas for conservation efforts.
- The Rise of Citizen Science: Machine learning can empower everyday citizens to contribute to scientific research. Imagine projects where volunteers collect data using smartphone apps, and machine learning algorithms analyze that data to solve problems like air pollution or wildlife conservation.